New research carried out by the Department of Energy’s SLAC National Accelerator Laboratory and Stanford University has demonstrated how neural networks can analyze complex gravitational lenses accurately millions of times faster than traditional methods. “Analyses that typically take weeks to months to complete, that require the input of experts and that are computationally demanding, can be done by neural nets within a fraction of a second, in a fully automated way and, in principle, on a cell phone’s computer chip, said co-author of the study and postdoctoral fellow Laurence Perreault Levasseur.
The research involved using neural networks to analyze images of strong gravitational lensing where the far away galaxy appears distorted into rings and arcs by the gravity of such a large object. These distortions are what tell us how mass is distributed in space and how that balance can change over time. Previously, researchers would spend weeks, or sometimes months analyzing actual images of lenses compared with simulations of lensing models. But now, with the help of neural networks, this tedious process can be done in a matter of seconds.
In order to teach the neural networks what to look for researchers showed them around 500,000 different simulated images of various gravitational lenses. This lasted for about 1 day. After this period, the networks were capable of analyzing new lenses almost instantly with an accuracy just as good as traditional methods. “The neural networks we tested – three publicly available neural nets and one that we developed ourselves – were able to determine the properties of each lens, including how its mass was distributed and how much it magnified the image of the background galaxy,” confirmed Yashar Hezaveh, a NASA Hubble postdoctoral fellow at the Kavli Institute for Particle Astrophysics and Cosmology (KIPAC).
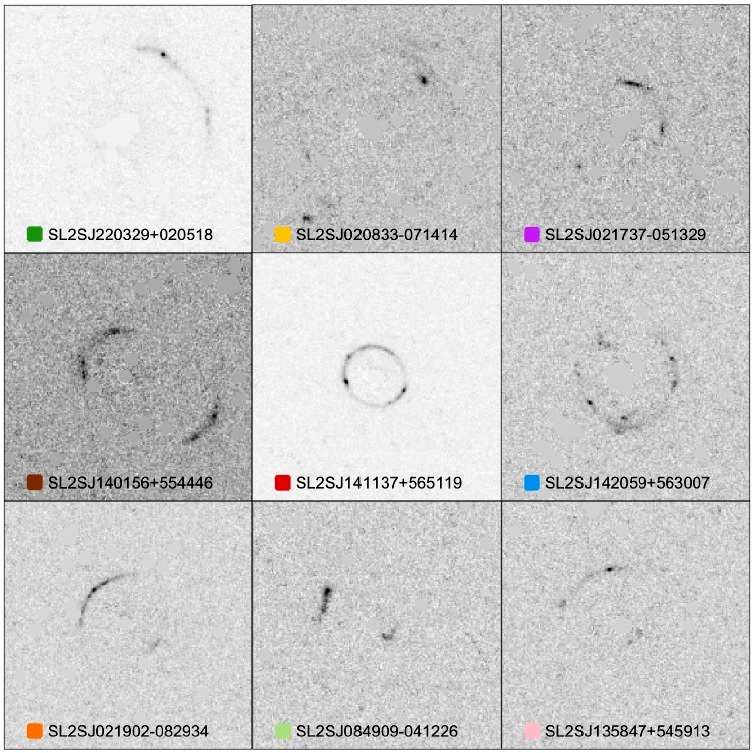
This is a giant leap forward in terms of what’s already in use with neural networks in astrophysics, which was predominately limited to solving mathematical equations. Being able to sort through huge amounts of data efficiently while performing complex analyses quickly in a fully automated fashion could change the world of astrophysics as we know it. Perreault Levasseur commented, “We won’t have enough people to analyze all these data in a timely manner with the traditional methods. Neural networks will help us identify interesting objects and analyze them quickly. This will give us more time to ask the right questions about the universe.”
The inspiration for neural networks come from the human brain where a dense network of neurons process and analyze information very quickly. In the artificial intelligence, version neurons are associated with the pixels of the particular image being analyzed. These neurons are made up of layers that look for features in the image. When layer one finds a certain feature, that information is sent to the next layers which then looks for another feature inside that one and so on and so forth. “The amazing thing is that neural networks learn by themselves what features to look for,” says Phil Marshall, KIPAC staff scientist and co-author of the paper. “This is comparable to the way small children learn to recognize objects. You don;t tell them exactly what a dog is; you just show them pictures of dogs.”
However, with artificial intelligence, it’s one step better than that because not only can the neural networks pick photos of dogs from the pile, but they can return information about the dog too, such as its height, weight, or age. And, according to KIPAC scientists, they could have run their computations from a laptop or cell phone had they wanted to. “Neural nets have been applied to astrophysical problems in the past with mixed outcomes, said KIPAC faculty member, Roger Blandford. “But new algorithms combined with modern graphics processing units, or GPU’s can produce extremely fast and reliable results, as the gravitational lens problem tackled in this paper dramatically demonstrates. There is considerable optimism that this will become the approach of choice for many more data processing and analysis problems in astrophysics and other fields.”
More News to Read
- Researchers Make a Breakthrough in Neutrino Behavior
- The Medical Implications Weightlessness Has on our Astronauts
- Researchers Discover Lonely Microbes Have Higher rate of Antibiotic Resistance
- Are Nanodiamonds the Answer to Safer Batteries?
- Scientist Identified a Portion of the Brain that Intensified the Desire For Cocaine