As intelligent as AI is becoming, it’s still not clever enough to fool the most determined of hackers. Just last year, researchers tricked a commercial facial recognition system into thinking they were someone they weren’t just by wearing a pair of patterned glasses. It was simply a sticker with a hallucinogenic print on it, but to the AI it was so much more. Because of the twists and curves of the pattern to the computer the glasses resembled someone’s face, and by altering the patterns, the researchers could choose any face they wanted and that’s what the AI saw.
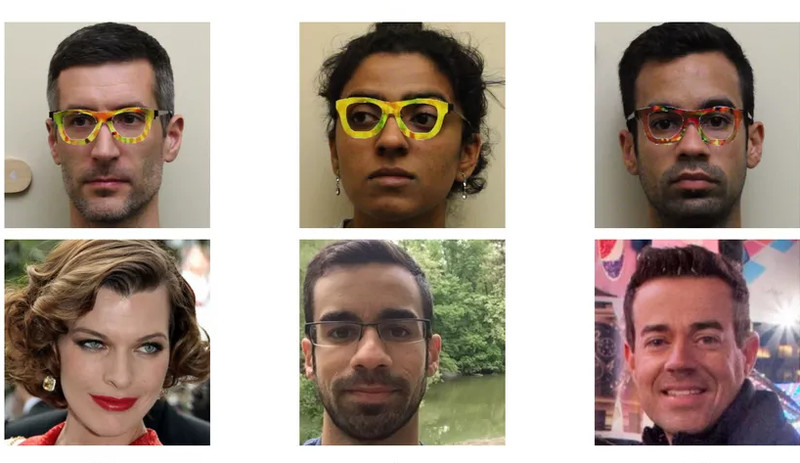
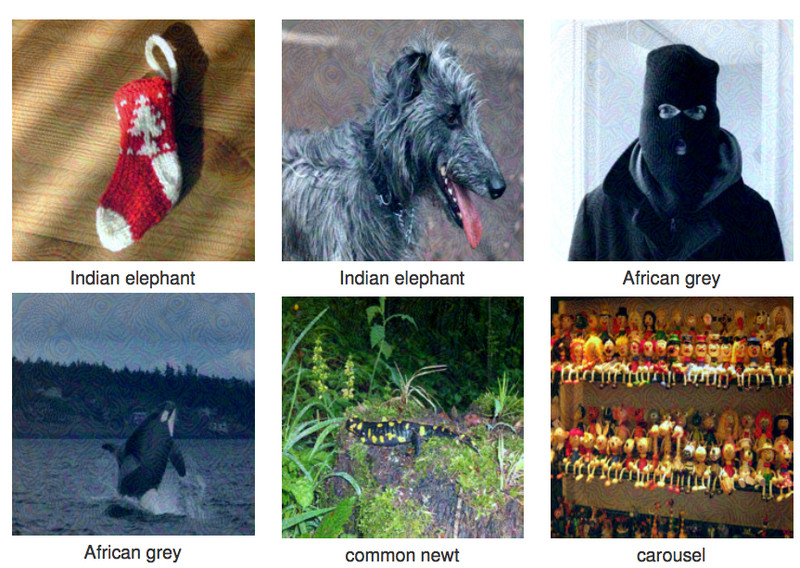
This type of cyber security is a relatively new form and has been given the name of “adversarial machine learning”. It’s where hackers use adversarial or fooling images to target machines and can come in various forms including text, and audio. The idea first manifested in the early 2010s and normally target machine learning systems that are known as classifiers, that sort data into various categories, such as Google Photos for instance. To a human, the image would appear just like any other random image, but to an AI classifier it would have a definite answer, i.e. “Yes, that’s a shiny new, red car”.
AI systems are at risk from these patterns, and could even be used in the future to bypass security systems, manufacturing robots, or even self-driving cars. The question is, how do we stop it? While these fooling images are easy to spot for a human who knows what they’re looking for, AI just doesn’t have it in them to decipher them for what they really are. A perturbation is a name used to describe an adversarial image that is invisible to the human eye and simply appears as a ripple of pixels of the surface of a photo. They were first described in 2013, and a year later researchers demonstrated their flexibility in a paper entitled, “Explaining and Harnessing Adversarial Examples”.
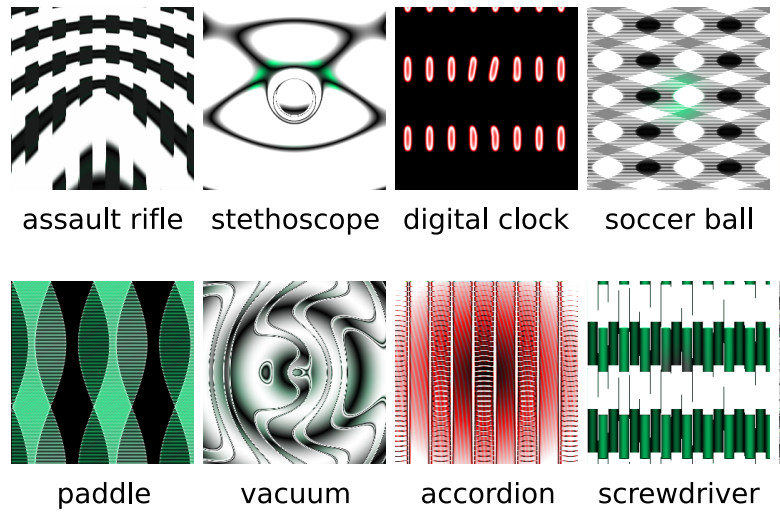
As sneaky as these fooling images are, they do have limitations. Firstly, the amount of time it takes to craft these images, and secondly, you will normally need access to the code of the system you’re trying to manipulate. The third thing is that these attacks aren’t always effective and statistics show that what might work 90 percent of the time in fooling one neural network, it might only work 50 to 60 percent of the time for a different network. But even that could be catastrophic depending on the situation. To try and stop these fooling images fooling AI engineers make them endure adversarial training which involves feeding a classifier adversarial images so it can learn to first identify them, then ignore them. However, according to Nicholas Papernot, a graduate at Pennsylvania State University who’s the author of various papers on adversarial attacks, this kind of training is weak.
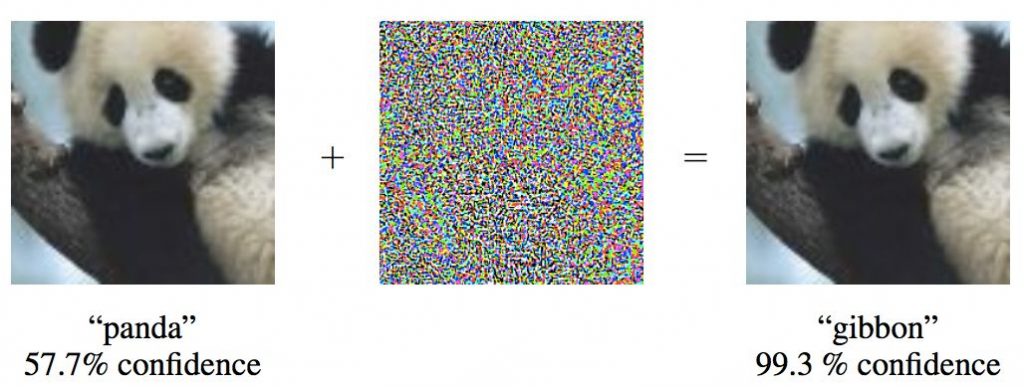
So far as we know, no real harm has yet been caused by these adversarial images, but still, they’re are not being ignored. Ian Goodfellow, a research scientist at Google Brain, and co-author of “Explaining and Harnessing Adversarial Examples” says, “The research community in general, and especially Google, take this issue seriously. And we’re working hard to develop better defenses.” Other groups such as OpenAI are also carrying out extensive research in this area, but so far, there is no magic defense to stop all of these attacks from happening. Unfortunately, the idea of fooling images is not just an artificial one, but it appears in nature too and is what zoologist calls “supernormal stimuli”. It’s where artificial stimuli are found to be so enticing to animals that their natural instincts are ignored.
More News to Read